Artificial Intelligence and the Changing Nature of Hiring:
Investigating the Impact of Perceived Benefits and Drawbacks of AI in the HR Recruitment Process on Candidates' Emotional Responses
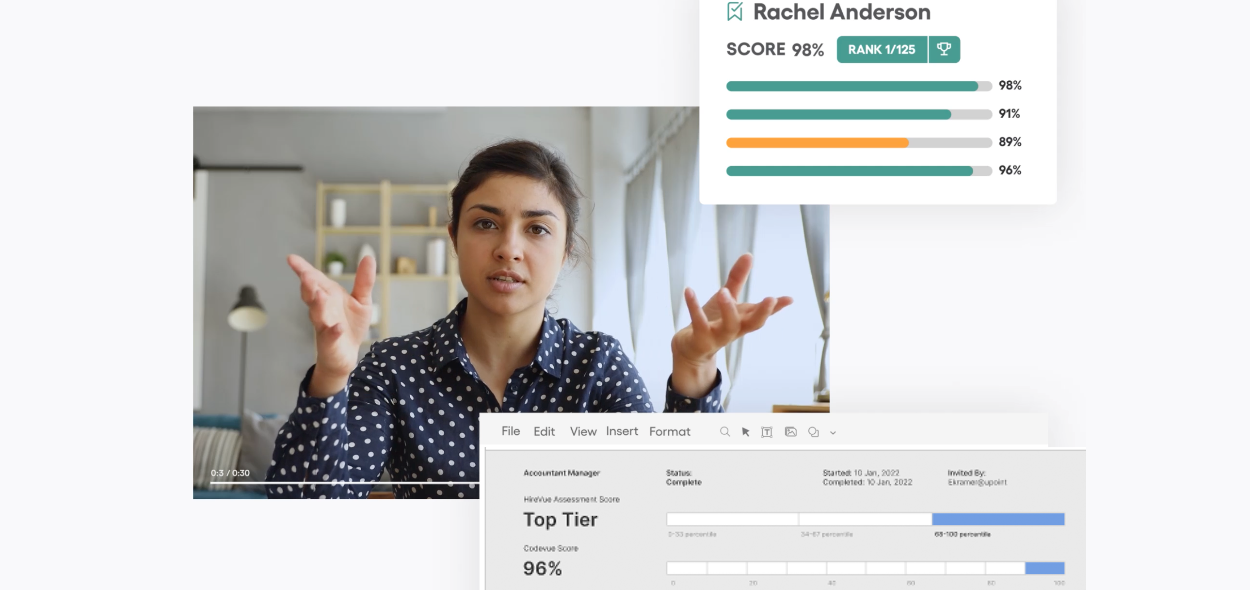
Topic
The rapid advancement of artificial intelligence (AI) technologies has recently attracted considerable attention and enthusiasm across various industries, including human resources (HR). AI-enabled recruitment tools can optimize and partially automate the selection process of applicants. This technology is able to analyze candidates' gestures, vocal tones, and facial expressions and thereby identify talents that qualify for a specific job opening. However, as AI continues to permeate the HR domain, a critical aspect that remains underexplored is the emotional impact on job candidates and how humans feel about AI in business life. Through the limited research on candidates' emotional responses, this work addresses and fills an initial research gap.
Relevance
Candidates' emotional responses can significantly influence their perceptions of the hiring organization, likelihood of accepting an employment offer, and future job performance. Furthermore, positive candidate experiences can enhance an organization's employer brand and reputation in the job market, making it more attractive to potential employees. As AI continues to transform the HR landscape, organizations must not only focus on the technical aspects of AI-driven recruitment processes but also consider the emotional and psychological impact on job candidates. This holistic approach is essential for designing recruitment strategies that foster positive emotional responses, design more effective AI-driven systems, enhance the candidate experience, and ultimately attract and retain top talents.
Results
AI recruitment systems are perceived as more objective, fairer, and more efficient than in traditional recruitment methods. However, such systems also exhibit reduced transparency, making them less comprehensible and dehumanizing the recruitment process. Based on the candidates' perceived benefits and drawbacks of such AI systems, positive emotions are reduced, and negative emotions are enhanced. Notably, negative emotions, such as disgust and sadness, as well as positive emotions, such as joy and hope, are prominent. What became apparent from the results is that a greater sense of objectivity, efficiency, transparency, and a natural and human process can increase positive emotions.
Implications for practitioners
• Companies must be aware that an algorithm aversion exists among job candidates from the lack of or insufficient transparency, the black-box character of such AI systems, and the lack of a human component. Appropriate ways of reducing this aversion must be found by making such systems more explicable and human.
• Organizations should invest in the skills and abilities of employees using AI-driven recruitment instead of hiring headhunters. Nevertheless, it is vital to ensure that the data used in the selection process are not biased or unfairly judged and that the decision-making process is not negatively impacted by outdated data.
• Managers and decision-makers should be aware that different AI recruitment tools and application areas can trigger different emotions in candidates depending on the scope and integration of the application. The selection and implementation of such programs must be devised and evaluated. In particular, solutions should be found to reduce negative emotions such as sadness, disgust, or even fear.
Methods
Through a literature review, perceived benefits and drawbacks of using AI in recruiting versus traditional methods were identified, which have an impact on positive and negative emotions. A quantitative empirical research approach was chosen to determine cause and effect and correlations and ensure the comparability of the results. A between-subjects experiment was designed using a self-reported online questionnaire. There were two groups (control and treatment), each with either a traditional or AI scenario. A total of 144 participants took part in the survey. For the analyses, correlation models as well as simple and multiple linear regression were used, which examined the different variables in order to test the hypotheses.