Exploring Practical Barriers to the Adoption of AI-based Application in Swiss Healthcare
This study addresses the barriers to artificial intelligence (AI) adoption in the Swiss healthcare system. AI revolutionizes diagnostic procedures and treatment options for patients, but restrictions put on it by the law and resistance from different stakeholders are hindrances to its adoption.
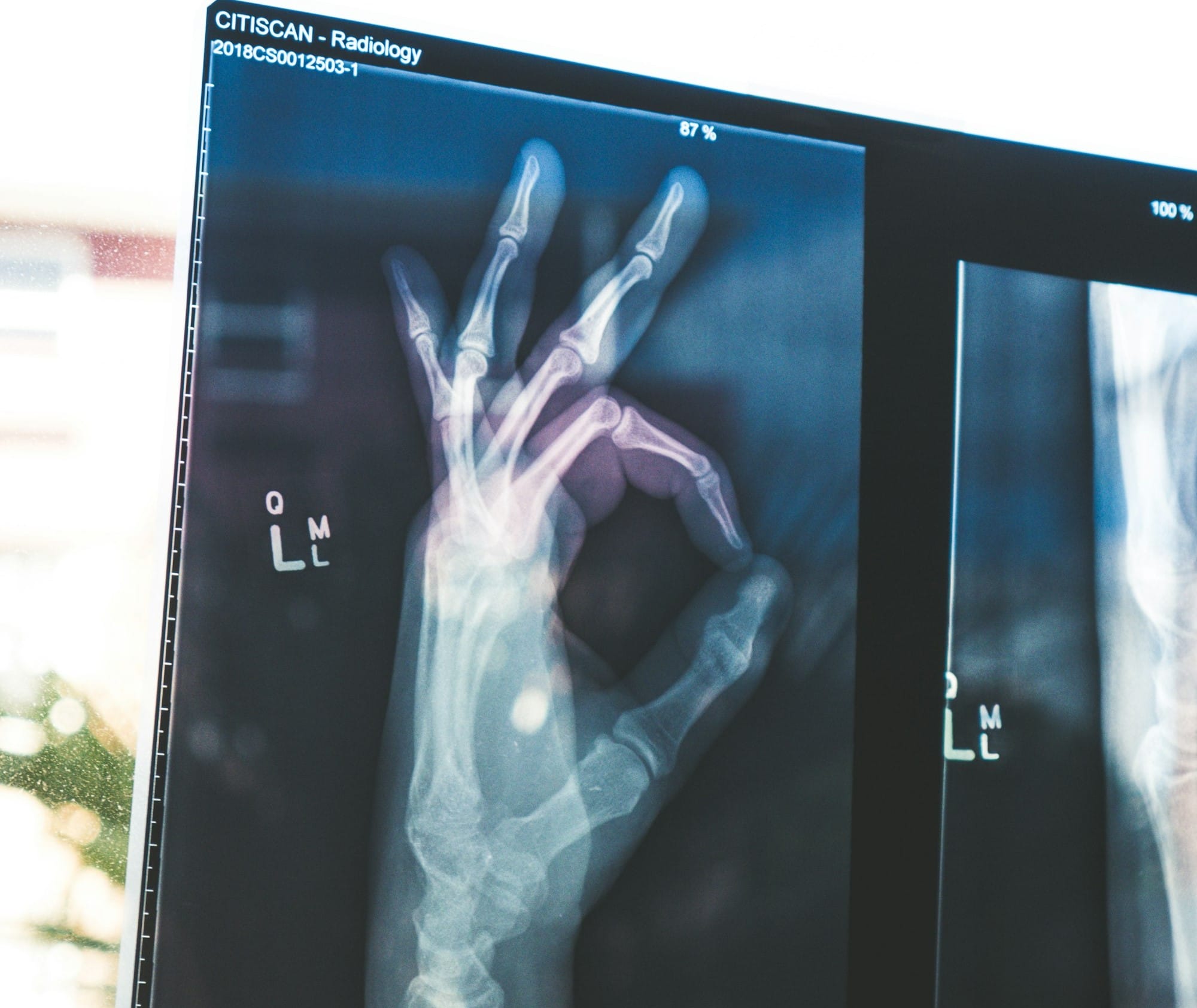
Topic
This study addresses the barriers to artificial intelligence (AI) adoption in the Swiss healthcare system. AI revolutionizes diagnostic procedures and treatment options for patients, but restrictions put on it by the law and resistance from different stakeholders are hindrances to its adoption. The outcome of interviews reveals that some major issues like regulations, financial aspects, and collaborations should be addressed. A comprehensive approach is characterized by stakeholders working together, enough financial support, and continuous evaluation. Bridging the gap between theoretical potential and real benefit in practice is an important factor in determining the potential of AI.
Relevance
The generation of large datasets in healthcare is producing clinically and financially meaningful data. AI can develop personalized medical predictive models that go beyond guideline-based treatments to individual patient care. As a result, there is a need for this change due to the increase in administrative efforts brought about by large amounts of patient data that doctors must process. There are many other instances showing how AI matters in health care, for example detecting pneumonia and recognizing skin cancer. The literature promises customized and well targeted therapeutic interventions that will improve quality of care. Nevertheless, adoption in Switzerland remains slow.
Results
Five main areas of focus were identified: Regulatory Framework, Perception of AI, Interdependencies, Financing Innovations and Collaborative Integration These indicate the challenges facing AI adoption. The existing laws are not sufficient to handle the unpredictable nature of AI and they should be reworked. Implementation requires changes in culture, the participation of stakeholders and data sharing mechanisms. Also, financing AI innovation is hindered by investment uncertainty and complex and expensive IT-infrastructure. So called sandboxes, which involve collaboration, are essential for adoption. To make step-by-step implementation possible in the healthcare system, these areas need to be tackled by the responsible stakeholders.
Implications for practitioners
- To enable AI use, regulatory frameworks must support outcome-based approaches and allow the generation of experience.
- Essential for healthcare institutions are collaborative funding mechanisms and shared infrastructure; these require a national or regional approach within canton structures.
- Theoretical value and practical effect should be evaluated by real-world implementation to gather experience.
- Dealing with trust problems and misconceptions about adopting AI requires time and experience to implement meaningful change.
- Aligning political interests among the stakeholders requires an open error culture and the willingness to experiment in all health care stakeholders to promote the experiential learning process.
Methods
This study employs an exploratory research approach, utilizing semi-structured interviews to examine AI adoption in Swiss healthcare. A literature review identifies current hurdles in AI and technology adaptation within the healthcare sector. Thematic analysis guides the investigation, with interviews involving stakeholders from organizational management, IT, medical device regulation, and government sectors. The use case of stroke recognition provides an illustrative example to guide discussions and highlight potential applications. Data analysis includes transcribing interviews and visualizing themes, as well as employing the macro-meso-micro model to highlight implications for various stakeholder groups.