Generative AI for Research Support: A Taxonomy
This master's thesis explores how generative AI can enhance scientific research by developing a taxonomy. Covering tasks, people, technology, structure, and ethics, the study highlights AI's potential to automate tasks, facilitate collaboration, and address ethical concerns in research.
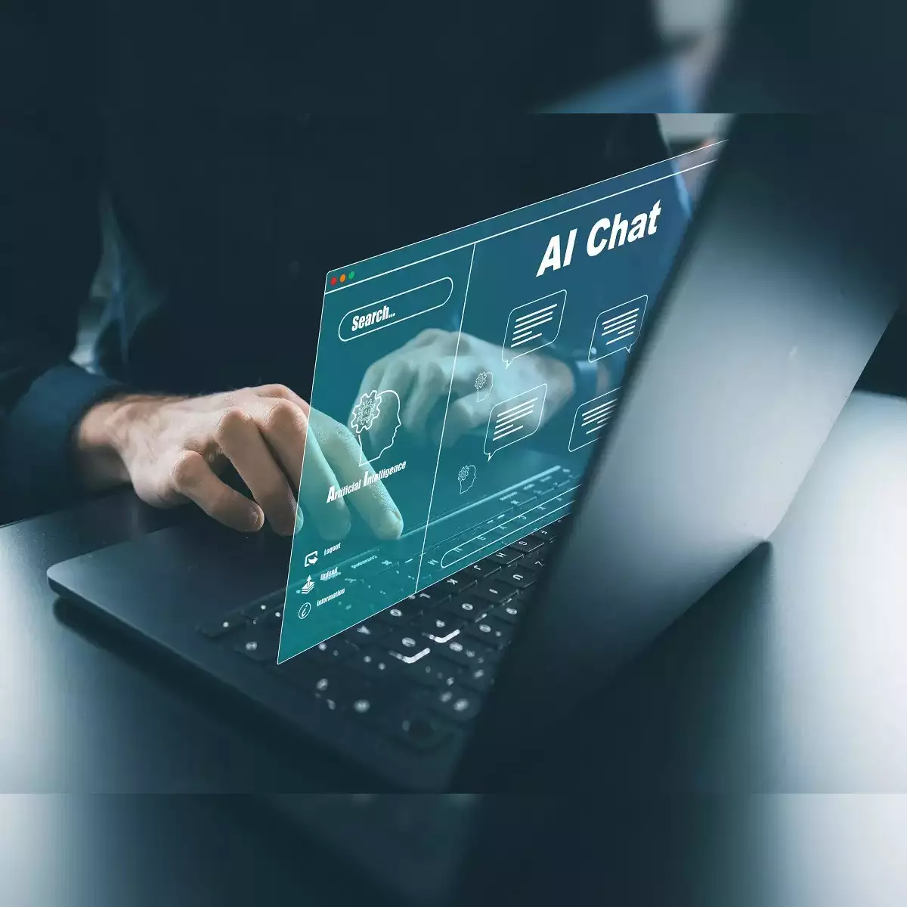
Topic
This master's thesis explores the integration of generative AI in scientific research, aiming to develop a comprehensive taxonomy that categorizes its diverse applications and impacts. The taxonomy encompasses five critical dimensions: Task, People, Technology, Structure, and Ethics, providing a structured and detailed framework for understanding how AI can enhance various research methodologies and outputs. By examining the roles and functions of generative AI, the study seeks to highlight the transformative potential of these tools in academic settings.
Relevance
The relevance of this topic is profound for practitioners and academics alike, as generative AI holds the potential to revolutionize research practices. Integrating AI into research can significantly enhance efficiency by automating time-consuming tasks, thereby allowing researchers to focus on innovative and analytical aspects of their work. Additionally, AI tools can facilitate seamless interdisciplinary collaboration and communication, essential for complex research projects. Understanding and addressing the ethical implications of AI use is crucial to maintain integrity, transparency, and trust in academic and scientific outputs.
Results
The study found that generative AI tools, such as ChatGPT, can substantially enhance research efficiency by automating routine tasks like literature reviews, data analysis, and hypothesis generation. These tools also facilitate interdisciplinary collaboration by enabling more effective communication and data sharing across research teams. Moreover, the study highlighted the economic benefits of AI in accelerating innovation cycles. However, significant ethical concerns, such as biases in AI-generated content and the need for transparency, were also identified, necessitating the development of robust ethical guidelines for AI deployment in research.
Implications for practitioners
- Efficiency Enhancement: Automate routine and repetitive research tasks such as literature reviews, data collection, and preliminary analysis, freeing up researchers to focus on more complex and creative aspects.
- Collaboration Facilitation: Use AI tools to enhance communication and data sharing across interdisciplinary research teams, improving collaborative outcomes.
- Innovation Acceleration: Leverage AI to speed up various stages of the research process, from hypothesis generation to data analysis, reducing time-to-publication and fostering quicker innovation cycles.
- Ethical Considerations: Implement comprehensive ethical guidelines to address biases, ensure transparency, and maintain integrity in AI-generated research outputs.
- Training and Awareness: Provide training for researchers on the capabilities, limitations, and ethical use of generative AI to promote responsible and effective adoption of these technologies in academic research.
Methods
The methodology involved conducting a systematic literature review to create a comprehensive database of existing generative AI applications in research. Following this, an iterative approach inspired by Nickerson et al. (2013) was employed to develop and refine the taxonomy. This approach included identifying meta-characteristics, developing dimensional items, and engaging in expert evaluations. Feedback from experts ensured the taxonomy’s relevance and applicability. The final framework categorizes AI applications across the dimensions of Task, People, Technology, Structure, and Ethics, providing a robust and practical tool for researchers to integrate generative AI effectively into their workflows. This structured and iterative methodology ensures that the taxonomy remains both theoretically sound and practically useful.