Maximizing Data Insights from MES for Discrete Manufacturing
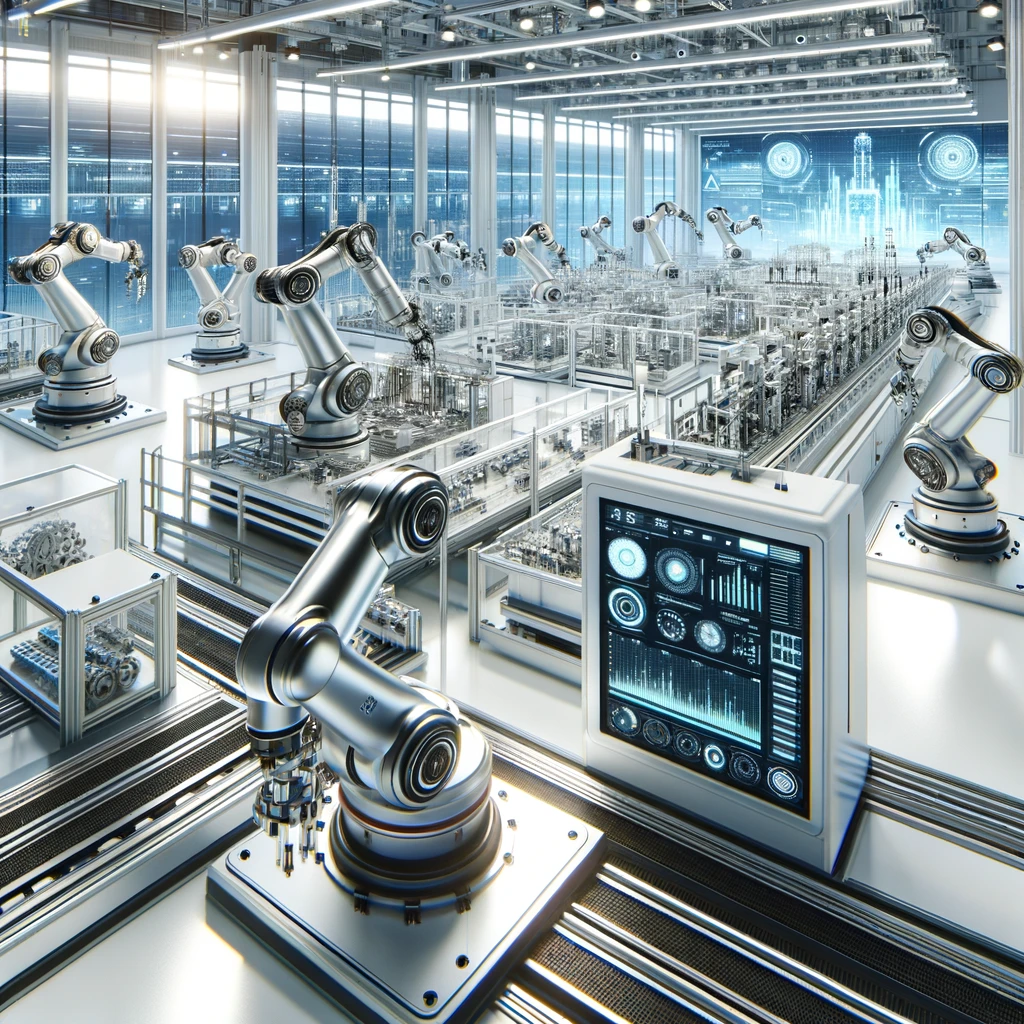
Topic
This master's thesis explores the development of a Data Quality Framework tailored for Manufacturing Execution Systems (MES) in discrete manufacturing. The study aims to improve the accuracy, reliability, and applicability of MES data by addressing challenges in data quality management and integration, which are critical for operational excellence and strategic decision-making in manufacturing industries.
Relevance
The relevance of enhancing MES data quality in discrete manufacturing is significant, as it directly impacts the operational effectiveness, efficiency, and adaptability of manufacturing processes. With the increasing integration of Industry 4.0 technologies, ensuring high-quality data is essential for manufacturers to remain competitive and harness the full potential of digital transformation.
Results
The research confirmed that a structured Data Quality Framework improves data handling, analysis, and decision-making within MES. Key findings include the identification of common data quality issues, the effectiveness of the proposed framework in real-world settings, and the utility of the Data Quality Assessment Tool, which was developed and validated as part of this study.
Implications for Practitioners
- Enhanced Decision-Making: Practitioners can achieve more accurate and timely decisions with improved data quality.
- Increased Operational Efficiency: Reliable data quality minimizes errors and downtime, enhancing overall productivity.
- Adaptability to Industry 4.0: A robust data quality framework supports the integration of advanced technologies like IoT and AI.
- Competitive Advantage: High data integrity enables better compliance with industry standards and customer expectations.
Methods
The methodology encompassed a mixed-methods approach, integrating both qualitative and quantitative research techniques. It began with a comprehensive literature review to define current standards and identify gaps. This was followed by the development of a proof-of-concept Data Quality Assessment Tool and an online survey to gather empirical data from MES users. The study utilized statistical analysis to validate the tool and framework, ensuring practical relevance and applicability.