AI for Predictive Maintenance: Driving Operational Efficiency and ESG Goals in Wind Energy Production
This study examines how AI-based PdM can improve operational efficiency and support ESG goals in wind energy. Using a literature review and expert interviews, it identifies key challenges and highlights PdM’s strategic potential for sustainable energy operations.
jacob.nigsch@students.bfh.ch
May 18, 2025
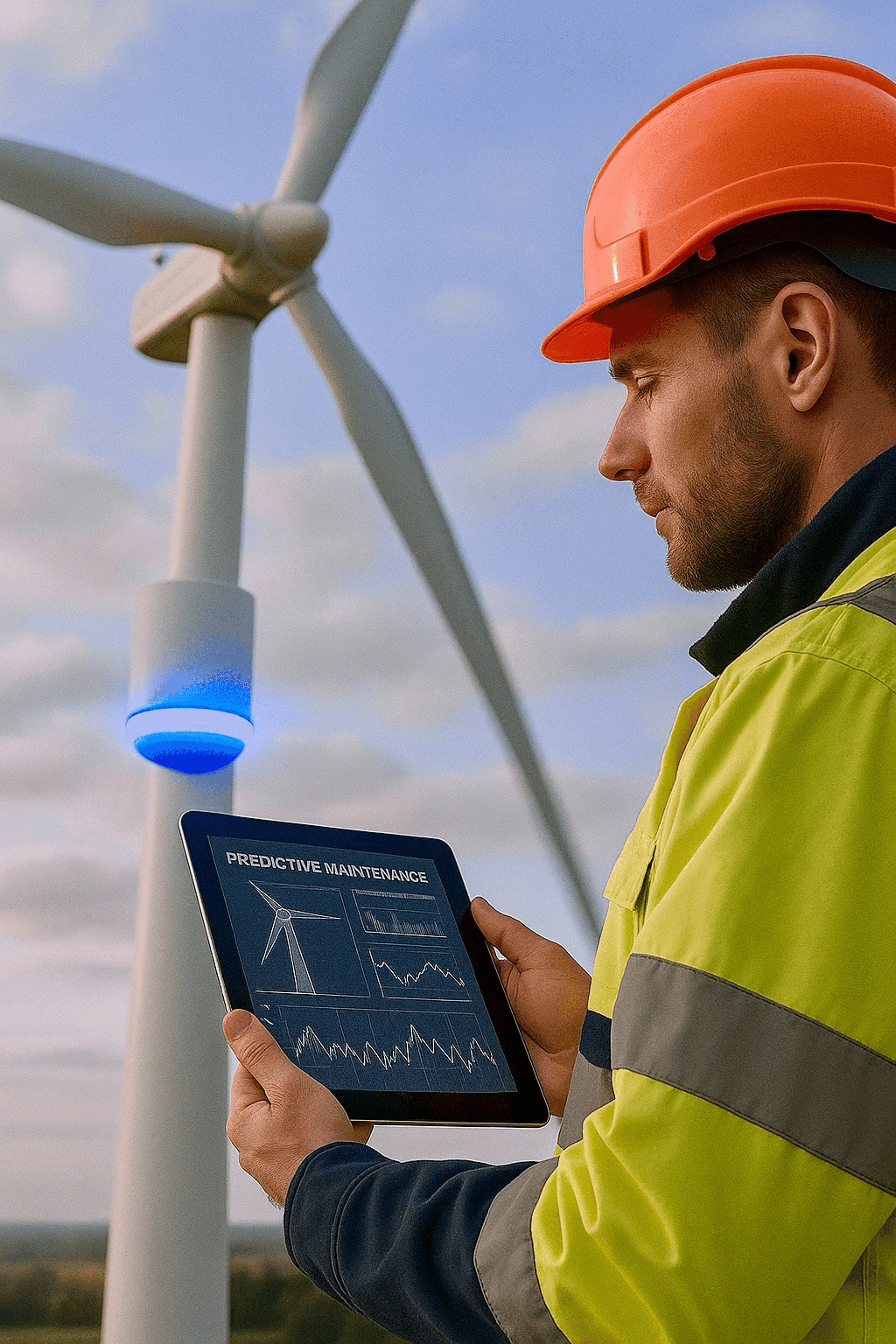
Topic
This study investigates how AI-supported Predictive Maintenance (PdM) can enhance operational efficiency while promoting ESG goals in wind energy production. The study identifies key benefits, challenges, and adoption factors relevant for the sector’s sustainable digital transformation.
Relevance
The integration of AI-supported PdM in wind energy operations offers promising opportunities to improve both asset performance and sustainability practices. While ESG criteria are gaining importance across the energy sector, practical implementation remains complex. This study contributes to a better understanding of how PdM technologies can support long-term operational goals and environmental performance, while also highlighting challenges related to system integration, data quality, and organizational readiness. It provides actionable guidance for decision-makers seeking to align PdM initiatives with ESG objectives and long-term asset performance strategies.
Results
The study shows that AI-enabled PdM can create value across all ESG dimensions:
- Environmental: Reduced emissions, extended component life, and resource efficiency
- Social: Improved worker safety, fewer high-risk inspections, and better workload management
- Governance: Enhanced traceability, audit readiness, and decision transparency
However, technological benefits alone are not enough. Successful implementation depends on data quality, organizational readiness, and the human factor, including user acceptance, cross-functional collaboration, and trust in AI recommendations. Explainability and transparency emerged as key enablers. The findings highlight the need to align technical design with human-centered integration and ESG strategies.
Implications for practitioners
- Position PdM as a strategic enabler by embedding it into broader digitalization and ESG transformation roadmaps – not as an isolated tool, but as an integrated component of long-term operational strategies.
- Build user trust and acceptance through explainable AI, intuitive dashboards, and transparent recommendations – especially critical in conservative, safety-driven field environments.
- Ensure organizational readiness by clearly defining responsibilities across leadership, operations, and ESG management. Establish dedicated roles or working groups for PdM integration, and ensure regular coordination between asset managers, technicians, and data teams.
- Invest in data quality and system harmonization, particularly across heterogeneous turbine fleets and legacy systems, to enable scalable and reliable PdM deployment.
- Use pilot projects to demonstrate value, reduce resistance, and build internal momentum – gradually evolving from reactive to predictive models and from isolated tool use to integrated decision support.
Methods
This study followed a qualitative research design based on the principles of Design Science Research. A Systematic Literature Review was conducted to establish a theoretical foundation on PdM, AI integration, and ESG alignment. To complement and validate these findings, twelve semi-structured expert interviews were carried out with professionals from the wind sector. The Technology Acceptance Model served as the analytical lens to evaluate adoption factors, while thematic coding was performed. The analysis combined deductive categories (e.g., PU, PEOU) with inductive themes such as trust, explainability, and integration challenges.